Table of Contents (click to expand)
Artificial intelligence is helping physicists working on particle accelerators in many ways. AI is being used to help manage the large volume of data produced by these experiments, to find patterns in this data, and to develop new ways of analyzing it. Additionally, AI is being used to develop new computer vision algorithms that can be used to detect features in particle jets and other data sets.
Experiments at the world’s largest particle accelerator, the LHC (the Large Hadron Collider), at the physics lab CERN, produces more than one million gigabytes of data every minute. Even after compressing this colossal amount of data, the data amassed in just a few hours is tantamount to an entire year’s worth of data that social networking sites like Facebook gather from users—far too much to save and study by mortals like us. This volume of data will also increase multifold in the years to come.
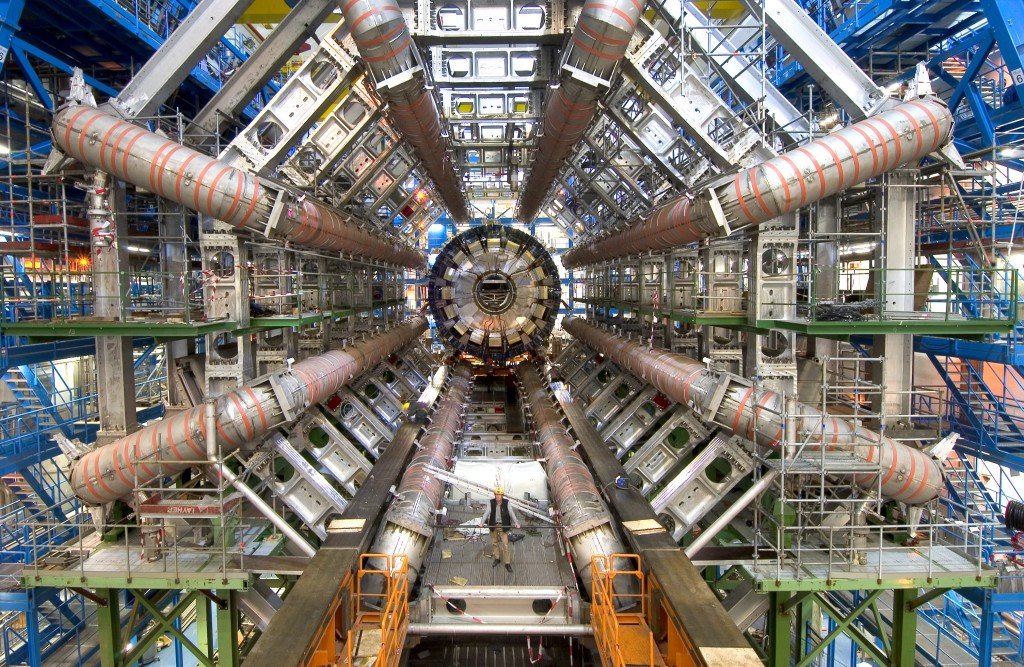
Driven by a conscientiousness to efficiently manage these discoveries with such staggering data volumes, physicists working on particle accelerators like the Large Hadron Collider (LHC) have brought in artificial intelligence (AI) experts to tackle the LHC data deluge. For the uninitiated, artificial intelligence (AI) is a specially designed set of software that mimics the way humans learn and solve complex problems. Machines running AI programs learn activities like speech recognition, planning, problem-solving, perception, and planning by themselves and can work efficiently without getting lost in the labyrinth of data.
The next generation of particle-collider experiments will engage some of the world’s most intelligent thinking machines if the links between particle physicists and AI researchers take off smoothly. Such machines could make incredible discoveries with nearly zero human input.
Recommended Video for you:
Role Of Artificial Intelligence (AI) In The Higgs Boson Discovery
Particle physics and artificial intelligence are not strangers. The ATLAS and CMS are two of the popular LHC experiments that paved the way for the discovery of the Higgs Boson a few years back. They were able to do so thanks to machine learning technology, a form of artificial intelligence that trains algorithms to recognize patterns in data and bring out meaningful conclusions from those patterns. The AI algorithms were primed using simulations of the debris from particle collisions and learned to accurately detect the patterns educed from the decay of rare Higgs particles among thousands of other unimportant bits of data.
Deep Learning
Recent advances in the field of artificial intelligence—often called ‘deep learning’—promise to take applications in particle accelerator even further. Deep learning uses structures that are loosely inspired by the human brain—consisting of a set of units (equivalent to neurons in our brain). Each unit combines a set of input values to generate an output value, which in turn is passed on to other neurons in the deeper layer of the network. In other words, deep learning refers to the use of neural networks—computer programs with a structure inspired by a dense network of neurons in the human brain.
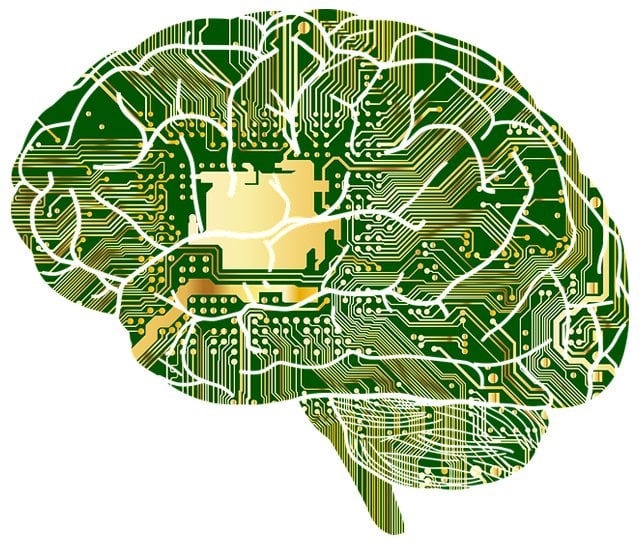
Rudimentary AI algorithms are trained with sample data, such as images, and are told (instructed) what each picture shows—a house versus a dog, for example. However, advanced ‘deep learning’ algorithms, such as those used by tech giants like Google (Google Assistant) and Apple (Siri) in their voice recognition systems, typically receive no such supervision and must find their own ways to categorize the data in their respective fields.
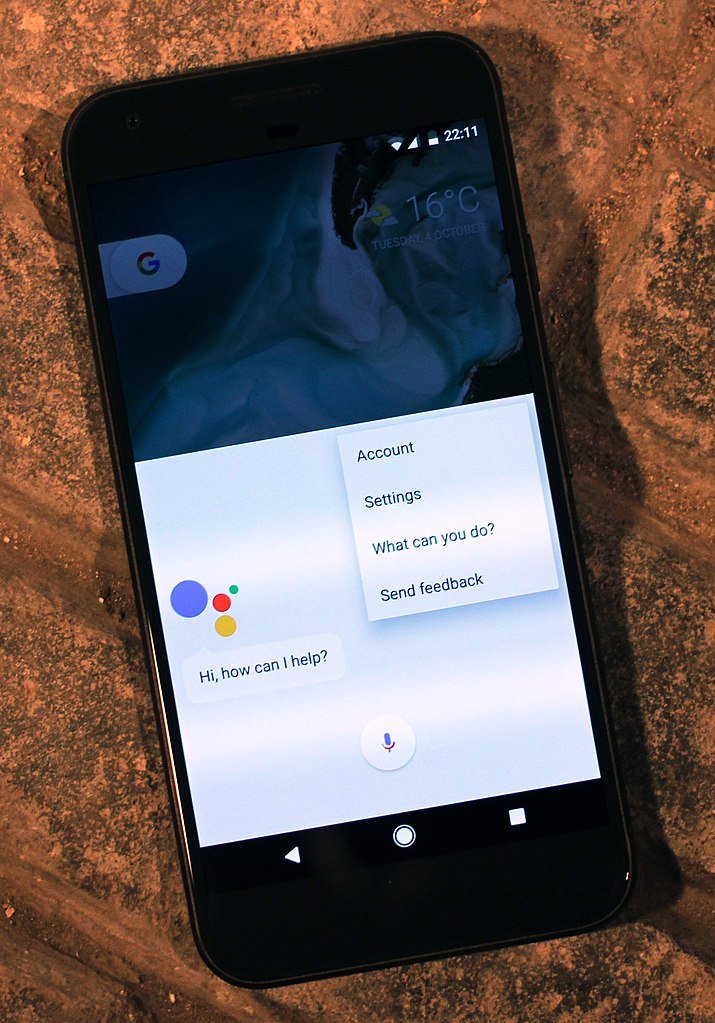
Until recently, the success of deep learning was limited because training the AI software to supervise themselves for subsequent uses was difficult. Also, earlier, the neural networks employed for deep learning were just a couple of layers deep, but thanks to recent advances in machine learning and neural networks, it is now possible to build and train networks that are thousands of layers deep. Deep Underground Neutrino Experiment (DUNE), an international mega-science project, employs these sophisticated deep learning algorithms to study neutrino science and proton decay.
Computer Vision
AI algorithms are becoming fine-tuned more and more each day, opening up fascinating opportunities to solve problems pertaining to particle physics. Many of the new tasks that AI programs use have their applications in computer vision. Computer vision deals with automatic extraction, analysis, and detection of relevant information from standalone images or a sequence of images. It’s similar to facial recognition, which most high-end camera phones come with these days, except that in particle physics, image features are much more abstract than simple facial features like your eyes, ears or nose.
Some popular neutrino experiments, like Nova and Microboone, produce data that can be easily translated into actual images. Computer vision algorithms can be readily used to discern features in such scenarios.
However, in particle accelerator experiments, images first need to be recreated from a heterogeneous pool of data that is generated by millions of sensor elements. Even if the data doesn’t look like images, physicists can still use computer vision programs if they process the data in the correct way, opines machine learning researcher Alexander Radovic.
One area where this approach could wield great results is in the analysis of particle jets produced in large numbers during particle accelerator experiments, like those at the LHC. Particle jets are narrow suds of particles whose individual tracks are extremely difficult to detect. Computer vision algorithms could help to identify features in jets.
Today, physicists are primarily employing artificial intelligence to find features in the large pool of data generated from particle acceleration experiments that can help us answer the biggest questions concerning particle physics. Ten years from now, AI algorithms might be able to independently ask their own questions and notify researchers when they come up with groundbreaking new discoveries in physics!
References (click to expand)
- Radovic, A., Williams, M., Rousseau, D., Kagan, M., Bonacorsi, D., Himmel, A., … Wongjirad, T. (2018, August). Machine learning at the energy and intensity frontiers of particle physics. Nature. Springer Science and Business Media LLC.
- CERN Data Centre passes the 200-petabyte milestone. The European Organization for Nuclear Research
- Higgs Boson Machine Learning Challenge | Kaggle. Kaggle